Excellence
- Home
- Overview
- Excellence
Rationale
The future needs data. Massive data that can allow future technologies, such as those based on AI and Robotics, to reach true utility, effectiveness, efficiency and usability for people, the world, and industries thereof. Currently, global surveys indicate that more than 50% of the companies are using AI technology (e.g., machine learning, computer vision, or natural language processing) in at least one business function, whereas more than 175 billion $ are invested annually in Artificial Intelligence as corporate investment[i].
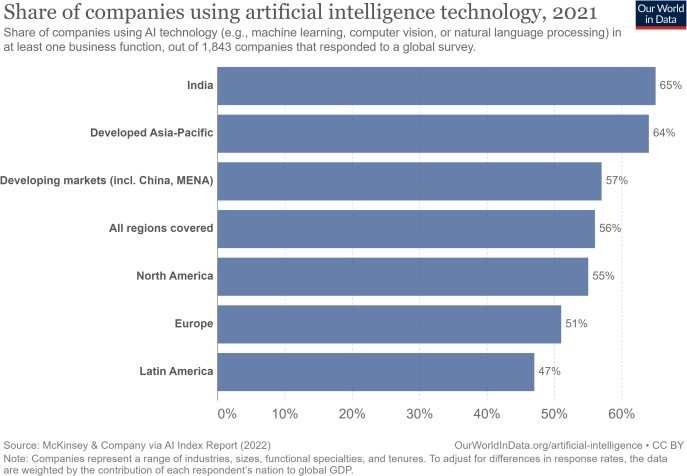
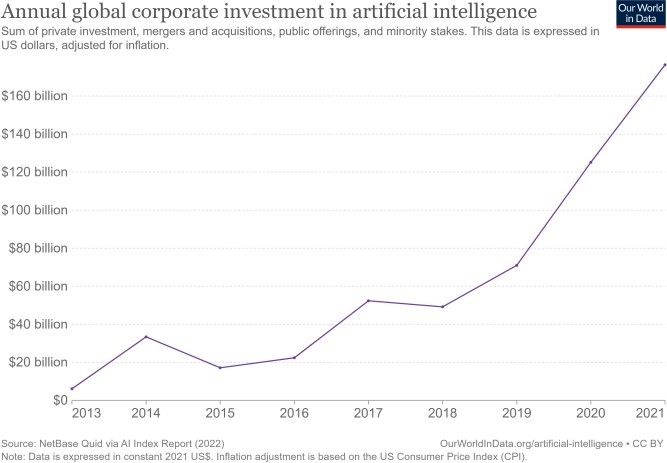
As AI technologies evolve, the need for massive amounts of real and simulated, yet realistic, data so as to further train machine-learning (ML) or deep-learning (DL) systems for real-world use, becomes more and more evident. Top results across technical benchmarks have increasingly relied on the use of extra training data to produce new state- of-the-art results. As of 2021, 9 state-of-the-art AI systems out of the 10 benchmarks featured in the 2021 AI Index Stantford report[i] are trained with extra data, implicitly favoring private sector entities with access to vast datasets. Smart and autonomous vehicles, robots that need to interact with operators and end users in healthcare and industrial settings, and AI optimized energy, circular economy and manufacturing processes, can all be substantially advanced through massive data that are taken from real operational settings and/or from digital twins and train ML and DL systems that are in turn, used in the real world providing further feedback streams of data.
Data spaces[i] provide a secure environment for businesses to exchange information with partners, customers, and stakeholders, which can lead to greater collaboration and outcomes inside the company departments but also, along data value chains. Access to high-quality data and the ability to use it effectively can be a crucial distinction for organizations operating in the European market in a continually changing industry. While data spaces are an important component of data management, they still do not deal with the entire data lifecycle and the interoperability between the different data spaces is still limited and with a lot of obstacles4. Although new initiatives like the ones from IDSA[ii], GAIA-X[iii] and SIMPL[iv] are making great steps towards data space interoperability, they are targeted more towards data sharing protocols and less into addressing the whole data lifecycle. The data lifecycle encompasses all stages of data generation and capture, as well as data transmission, storage, processing, analysis, and discarding. A comprehensive strategy to the data lifecycle is required to assure data quality, trustworthiness, security, and control, as well as to maximize its value. Failure to address the entire data lifecycle can result in data loss, abuse, and mismanagement, all of which can have major negative impact for EU competitiveness. In addition, while some basic integration of data spaces is already a possibility, a richer integration of data life cycles across data spaces and across domains could bring significant benefits for European competitiveness, including improved data quality and data integration, enhanced collaboration, increased efficiency, also in terms of energy used in whole data lifecycle and better data governance.
[i] https://internationaldataspaces.org/
[ii] https://internationaldataspaces.org/
[iv] https://digital-strategy.ec.europa.eu/en/news/simpl-cloud-edge-federations-and-data-spaces-made-simple
Specific Needs and Challenges
Multiple European initiatives concerning data space interoperability[i] aim to create frameworks for seamless data exchange across different domains, sectors, and Member States. However, some of the challenges that the EU data space interoperability initiatives still face include:
- Data Quality and Standardization: Data interoperability requires consistent data quality and standardization across different domains and sectors. This can be challenging as data is generated and managed by different organizations with varying data structures, formats, and standards, while are also frequently subject to human factors.
- Data Governance: Effective data governance is essential to ensure data privacy, security, and compliance with data protection laws. It requires setting up policies and procedures to manage data access, sharing, and usage across different domains, sectors, and Member States.
- Data Integration and Interoperability: Data integration is the process of combining data from different sources to create a unified view. However, data integration can be complex due to differences in data structures, formats, and protocols. Effective interoperability requires developing common data standards, formats, and protocols that enable seamless data exchange.
- Data Analytics and Visualization: Analytics and visualization tools are essential for deriving insights and making informed decisions from data. The availability of such tools can vary across different domains and sectors. Effective data space interoperability requires ensuring that analytics and visualization tools are available and accessible to all parties concerned, counteracting as necessary for human factors.
- Data Preservation and Access: Data preservation and access are critical for ensuring that data is available and accessible for future use. However, preserving and accessing data can be challenging as data is generated and managed by different organizations with varying data storage systems and formats. Most initiatives fail to address the challenges that arise in the full data lifecycle, from data generation and acquisition to storage, processing, analysis, sharing and disposal.
[i] Data economy: an open data market unleashing the untapped potential of SMEs – European DIGITAL SME Alliance
Opportunity
The current state of data management presents a significant opportunity for EU organizations across different domains affected by the progress in AI and Robotics, to differentiate themselves in the market and increase their competitiveness through the advancement of the state of art in data spaces. In today’s digital age, data is a key asset, and the ability to acquire, analyze, and utilize it efficiently may provide firms a decisive advantage. Even though data spaces provide a secure environment for organizations to communicate data with partners, consumers, and stakeholders, their interoperability is still limited, and there are several challenges to overcome. In response to these issues, the EU has launched several efforts, such as IDSA, GAIA-X, and SIMPL, to encourage data sharing protocols and improve interoperability between data spaces. To maintain data quality, trustworthiness, security, and control, and to maximize its value, a complete plan covering the full data lifecycle is still necessary. Through these initiatives, the importance of further data integration and full data lifetime consideration is clearly underlined.
While data spaces are an important component of data management, they still do not deal with the entire data lifecycle. Essential integration of data spaces is already a possibility, however, a richer integration of data life cycles across data spaces and across domains could bring significant benefits for European competitiveness, including improved data quality and data integration, enhanced collaboration, increased efficiency and better data governance, along with paving the way for step changes towards further advanced AI and Robotics technologies, both in terms of effectiveness and efficiency as well as of human-machine and more holistic human-technology interaction.
PLIADES targeted breakthrough
The project will research and develop a novel data integration framework that builds on key SoA architectures, and extends them with a series of advanced elements that solve essential, complex, yet practical problems around data green creation and storage, ownership and discovery, as well as use, re- use and disposal, among diverse data spaces. The project framework will be deployed on six use cases of advanced technologies that span among five data spaces; i.e. among the
- mobility,
- healthcare,
- green deal/ circular economy,
- energy and
- industrial data spaces.
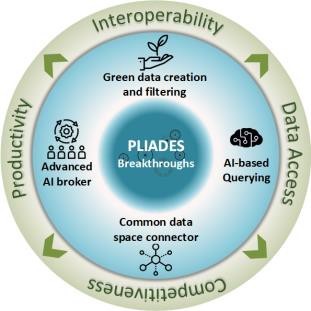
PLIADES connects EU data spaces, and advance state-of-the- art data management ideas. The project will expand data spaces to span complete data lifecycles, analyze them for greater understanding, while AI-based integration shall link and update them. Smart brokers shall propose data spaces based on a person or organization’s data needs. The developed smart brokers will be capable to understand request contexts and make suitable suggestions based on ML. This strategy will save time and money by allowing users to search numerous inter-connected, yet distributed and sovereign data sources. The project will also design and validate a training and support approach to help individuals, corporations, and organizations adopt the new standards and architectures, easing the transition to the new data landscape. It will foster innovation and fresh insights by combining data from several sources, boosting EU economic development and quality of life. The goal is to validate the proposed data space integration and augmented management approach by building meaningful data space aggregations in the aforementioned domains, proving its ability to provide insights and improve decision-making, and analyzing the viability of the suggested integration technique and whether businesses, industries, and organizations of various sizes can easily adopt it.